Behind the Music Recommendation Curtain: Computing Taste with Nick Seaver
In this conversation, Allison Jerzak spoke with cultural anthropologist Nick Seaver, who studies the intersection of people, technology, and culture. His 2022 book, Computing Taste: Algorithms and the Makers of Music Recommendation, draws on several years of ethnographic fieldwork at music recommendation companies in the United States.
A transcript is provided for increased accessibility.
Seaver’s project examines the people who create music recommendation systems: how they think about different kinds of listeners, how algorithmic recommendation creates new ways of listening, and the abstract, digital musical “spaces” that these systems create. Seaver finds that developers enculturate music recommendation systems, “teaching” them to hear in a culturally specific, North American way — an enculturation at odds with these companies’ ostensible goal of creating recommendation systems which are “open to anything that might happen.” Consumers encountering algorithmically curated playlists on platforms such as Pandora or Spotify can now engage in a new kind of reflexivity: why is this song being played for me right now? What logic delivered this song to me? Ultimately, Seaver finds that systems often recommend music based on patterns of listening behavior, a radically different way of curating and consuming music than in the past.
Highlights from our conversation include:
- On historical resonances between nineteenth-century player piano technology and twenty-first century algorithmic systems: “One of the things that I wrote about when I was working on the player pianos was how [people] were trying to think about what was distinctively human or distinctively musical in relation to these technologies…I think what you see today, sort of unsurprisingly, are similar efforts to think about what’s human in and around these systems. Especially, I would say, with more recent stuff like generative AI, to start to imagine what the human is again in relation to what these systems can and can’t do.” (3:05)
- On musical taste: “What I found in the field was that people didn’t really have theories about taste. I expected them to have pretty strong theories. Instead, they had this real openness…Most of the technologies that they were building were aimed at trying to be ready for whatever might happen, in terms of taste. People might like music because of how it sounds; but they might like music because of what their friends like; or they might like music because of where they are or what they’re doing. And there are all sorts of influences that they wanted to try to be ready for, or to develop systems that were kind of generic and open enough to capture it.” (6:15–6:50)
- On listeners: “A very dominant model of listener variability in the field is usually glossed as lean-forward or lean-back…in general, the idea is that a lean-forward listener is someone who is actively looking for things—they’re searching for stuff, they’re clicking on things, they’re interacting with the interface. They’re willing to expend effort to find new music…The lean-back listener, by contrast, is listening to music in the background, and maybe does not want to put in so much effort to click and find things; maybe doesn’t even care as much about music.” (8:20)
- On acousmatic listening in algorithmic systems: “When you listen to music in a recommender system, there’s a different order of acousmatic listening happening. I’m referring to that experience—which is a peculiarly contemporary experience—of hearing a song and thinking not, ‘what’s the source of this song?,’ or [about] what instruments made it. But rather, ‘why is this song being played for me right now? What is the logic that delivered this song to me?’” (13:50)
- On testing music recommendation systems: “You can bring what you know into these systems. And because this happens all the time while people are developing these systems, there’s this kind of pervasive enculturation of the overall system that happens in a very casual way, just by the people who happen to be there.” (19:25)
- On developers’ self-reflexivity: “It’s easy for people who come from my corner of the university to imagine that…everybody else who works on this stuff is some sort of idiot and that they don’t know. They never thought about the fact that there’s a cultural bias based on their own experiences…Music recommendation is a domain where nobody can pretend that there’s not something cultural going on.” (20:20)
- On musical space: “The relationship between genres and clusters is an interesting one… clusters sort of take over, maybe, the role of genres in these systems. If you, like many people, at the end of the year, get your Spotify Unwrapped. They’ll say, ‘Here’s your favorite genres’ or whatever. And what are those? It will sometimes be a list of styles of music that you’ve never heard of before. Because what they are are names for clusters of some kind of coherent listening behavior.” (21:35)
- On pastoral metaphors and control in these systems: “When people use [pastoral] metaphors, they’re often talking about the amount of control they have, which they experience as real. They know that they can change things. The guy who designs the genre system at Spotify — he can change how that works. And he knows that. But, he also knows that he can’t do anything he wants… I think those metaphors usefully talk about the kind of confusion and intersection of control, arbitrariness, chaos, instrumentation, all of these nature, culture and technology things that these people encounter it in their work.” (27:50)
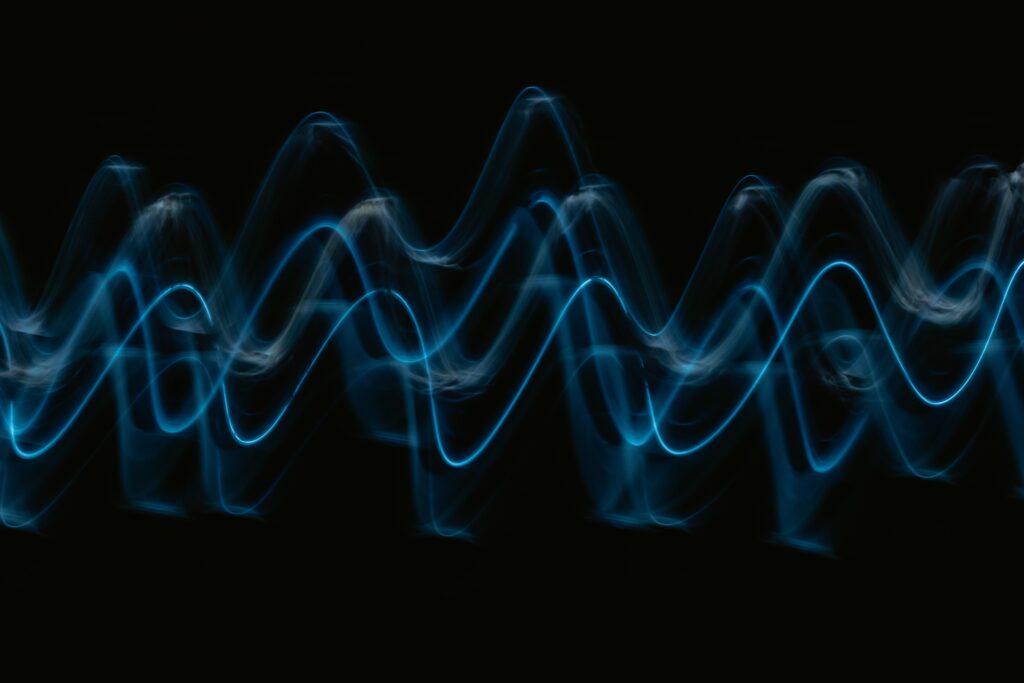
Image by Pawel Czerwinski (Credit: Unsplash)
Music credit: “Algorithms” by Chad Crouch.